Co-authored by Behzad Fatahi, Roger Hadgraft, Rosalie Goldsmith, and Fang Chen.
The disruptive impact of generative artificial intelligence (GenAI) has become a staple topic in educational discourse. Platforms like ChatGPT, now accessible to the public, epitomise how fast these tools are developing. For instance, ChatGPT’s utilisation in professional writing has generated significant attention (not all of it positive), showcasing AI’s potential in various domains. While the current GenAI platforms may lack competence in certain fields and include major errors in responses, it is evident that they are progressing rapidly, suggesting that accurate and reliable responses are not far off. A recent report by The Australian has detailed how the use of an AI-based in-house advice generator by Australia’s largest law firm allows tasks that used to take two hours to be completed in seconds. Given the pace and quality of these developments, the question emerges: how will human interaction with GenAI shape the future?
The transforming professional landscape and the crucial role of higher education
In many professional settings, tasks such as planning, investigation, analysis, and report preparation are undertaken by individuals, often followed by a review from a more experienced colleague. However, the rapid advancement of GenAI suggests there will be a shift towards initial problem analysis and report drafting being conducted by AI. This evolution points to a future where human professionals primarily assume the role of validators, verifying the accuracy and relevance of AI-generated content. This shift necessitates a re-evaluation of the skills required in the workforce, particularly in terms of validating and revising AI-generated outcomes.
Such a shift underscores the need for universities to adapt their curriculum to this emerging way of working with AI. Higher education must focus on teaching the fundamentals and technical principles that enable individuals to competently verify the outcomes of Gen AI. While it is beneficial for students to engage with and understand AI’s capabilities, it is equally crucial that they acquire technical skills from authoritative sources. Otherwise, we run the risk of seeing students becoming overly reliant on GenAI in their studies, and lacking the necessary evaluative skills to add value in industry settings.
The current generation of professionals, who have acquired their knowledge from traditional sources, plays a key role in validating AI outcomes. However, as these professionals retire, the new cohort, if primarily educated through AI, may lack the essential skills for validation, potentially leading to a crisis in the industry.
This is where the critical point lies, and universities have a pivotal role to play. They are responsible for training graduates who possess the fundamental knowledge necessary to progress into roles as verifiers as they advance professionally. Simply being proficient at prompting GenAI to produce outcomes is not sufficient for the future.
How to prepare for an AI-integrated future
While the technical concepts of advanced computer simulation software (e.g., solving complex mathematical equations using numerical techniques) and GenAI are fundamentally different, one may see the emergence of the latter mirroring the advancements in the former over the last 30-40 years, emphasising the necessity of developing validation skills in higher education. This need is further highlighted by the principle of Garbage in, Garbage out, which applies to both traditional software and GenAI. The quality of any software’s output is only as good as its input data. In the case of GenAI, the technology accesses a mix of reliable and unreliable data sources, meaning that conclusions could be influenced by less credible information. This reality makes the verification process critical, akin to the checks that users of advanced computer simulation software perform to ensure outputs are reliable and meaningful. Integrating this verification approach into academic assignments could bridge the gap between reliance on sophisticated technology and maintaining essential skills in critical evaluation and validation of AI outputs.
A multi-faceted approach
Thus, universities should invest in and utilise a multifaceted approach to teach students how to validate and determine the accuracy of information provided by GenAI systems. This can include:
- Learning about authoritative, proven, or best available techniques and software for analysis and design: For cross-referencing and validation, students need to learn how to compare information from AI with multiple reputable sources. This is particularly important for critical or sensitive information.
- Utilising case studies and real-world examples: GenAI might struggle with context and nuance, especially in complex or nuanced topics. It is important to assess whether the AI response adequately addresses the specific context of a query or report.
- Fostering collaborations with industry and academic experts: For specialised or technical information, students should learn to consult experts in the field. Professionals can provide context, depth, and accuracy that AI might not be able to match.
- Training in research methodologies and critical thinking: Due to the rapid expansion of knowledge, students need to be trained to conduct meaningful research and apply critical thinking to evaluate information, checking for consistency, logical coherence, and alignment with known facts.
- Emphasising verification of data sources: GenAI systems are trained on vast datasets but may not have access to real-time data or the ability to verify the sources of their training data. Therefore, teaching students how to cross-check information provided by GenAI with reliable and up-to-date sources is important.
- Understanding limitations: GenAI can sometimes produce plausible but incorrect or misleading information. Awareness of this limitation is crucial, especially in fields requiring up-to-date or highly specialised knowledge.
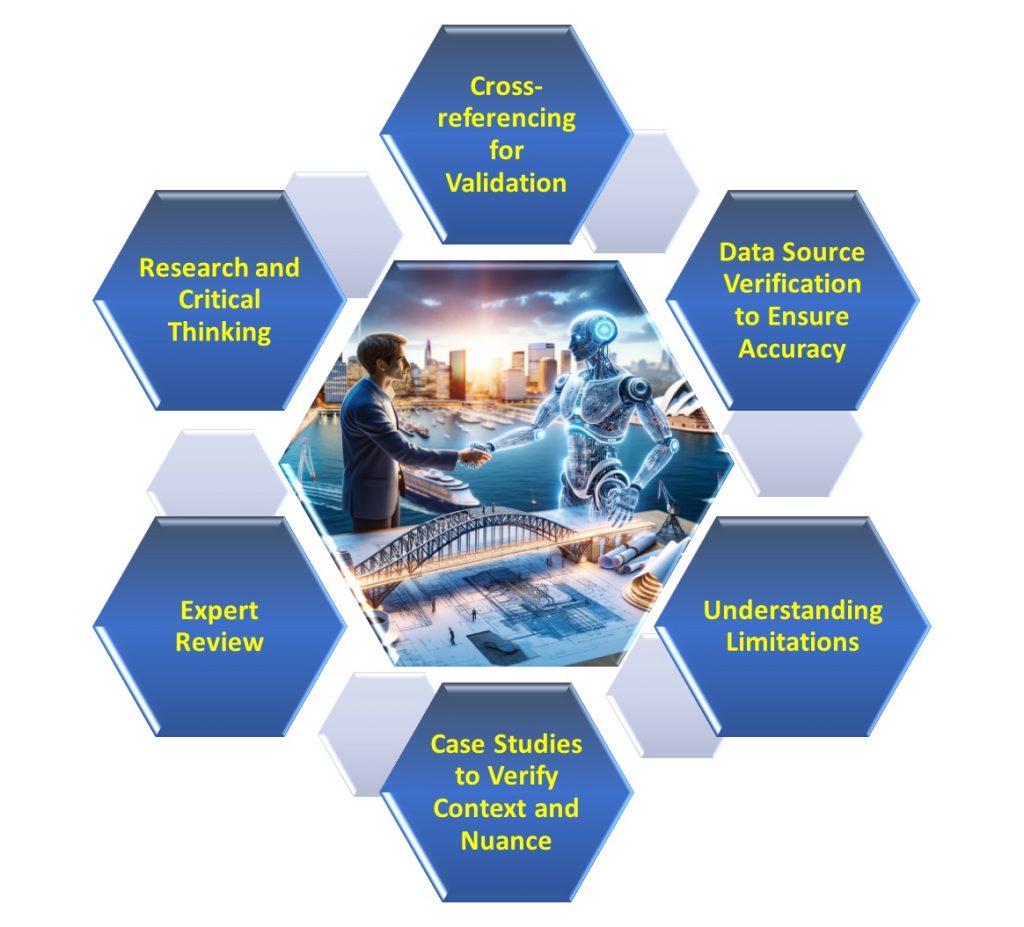
Equipping students with the right tools
These strategies collectively prepare students to critically assess and validate AI outcomes effectively in their future careers. The recent research study by Fatahi et al. (2023), which received the Best Research Paper Award at the 34th Australasian Association for Engineering Education Annual Conference, showcased examples of how university students can learn to validate GenAI using another independent source, such as rigorous computer simulation, while also enhancing their critical thinking skills. This is, of course, assuming that humans will still remain in charge and use GenAI as an assistive tool for the foreseeable future. We can use the analogy that the human is the pilot and the GenAI acts as a co-pilot. However, the day may arrive when AI can perform validations with reliability comparable to or surpassing human expertise, indicating a transition from human-led to AI-led validation processes. Such a scenario, while challenging to predict in terms of its occurrence, cannot be ruled out. It represents a critical juncture where humans might lose primary control over AI systems, especially as we witness the emergence of self-validating AI mechanisms. Until that day arrives, our focus should be on enhancing our skills in using and critically assessing AI tools. It is essential to develop a proficient workforce adept at validating AI outputs, ensuring that until AI can validate itself, we maintain the expertise and capability to effectively oversee and guide AI applications.
Let us all remember what Charles Darwin (1809 – 1882) said: “It is not the strongest of the species that survives, nor the most intelligent that survives. It is the one that is most adaptable to change”.
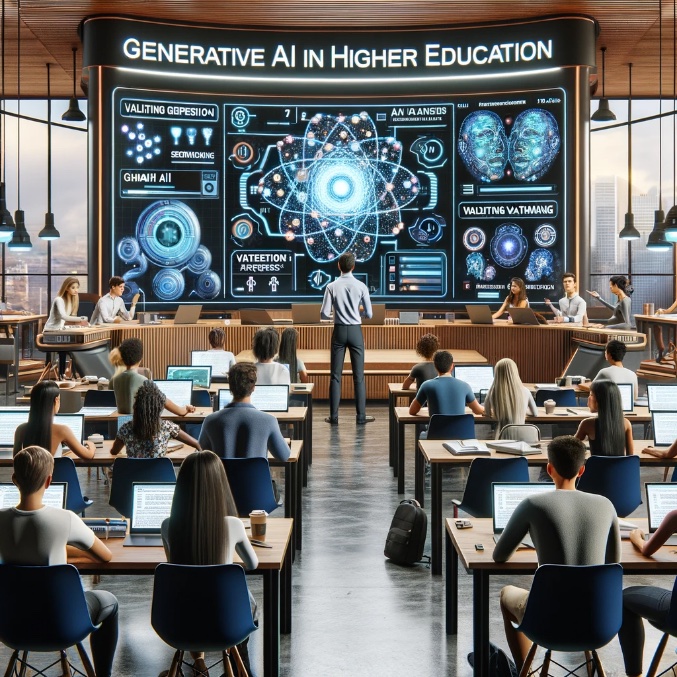